#ConnectionPGH Talk: “How To Apply Big Data To Your Work (Cheaply)” by Alison Alvarez
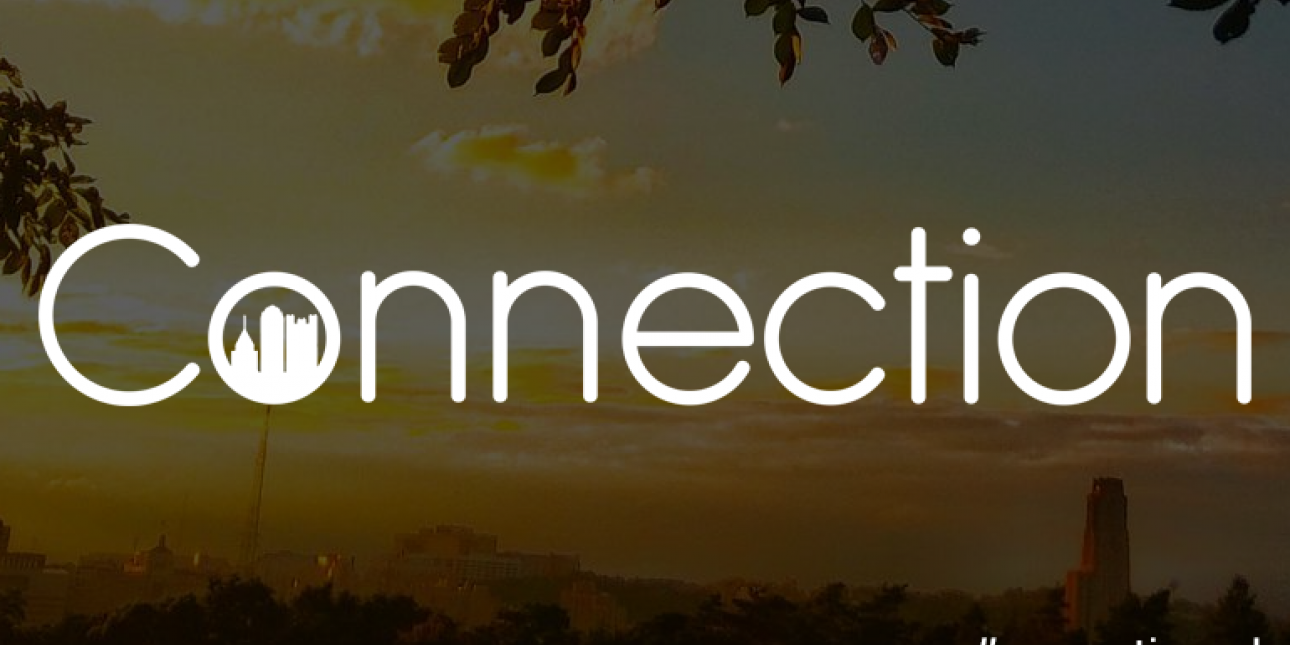
About The Speaker
Alison Alvarez is the CEO and co-founder of BlastPoint. She spent years building big data tools for large companies and noticed that there was nothing comparable for smaller organizations without data professionals. She built BlastPoint to address those needs by making data affordable and accessible through the magic of maps.
About Connection 2017
The Connection 2017 experience took place in Pittsburgh, PA. To learn more about the event, visit our Connection page today.
About The Talk
More and more, the term "big data" is thrown around in every industry imaginable, but what does it mean for youth-serving organizations? In this informative talk, Alison Alvarez details how you can use big data in your every day work (and do it cheaply!)
Transcript
I want to ask everybody a personal question: have you ever felt victimized by data in the course of your ordinary job? I mean, I think I have, yeah, yeah, we all feel like data is hard, isn't it? It's hard to find the right data, it's hard to figure out even if it is the right data once you've had it. It's hard to communicate it to other people, it's hard to keep it fresh, and that's because like a lot of the data that we get ends up looking like this. And you guys have, probably most of you, I've heard lots of people talking about the US Census. This was census data. So I'm a data scientist, I don't know how you guys feel looking at this kind of data, I feel overwhelmed and frustrated. Do you guys feel the same way? Yeah, yeah, but I want to say conversely that even though the stuff looks like it's a hard thing to start with, I think data is actually for everyone, and I know there are a ton of organizations represented here who use data in your everyday mission, use data to get grants, use data to figure out who you need to target, you've used data to figure out how to plan for the future. I worked for big corporations for a long time, and I learned a lot of different techniques that were used internally to help data make sense both internally within the staff, and externally, to whoever they were targeting. And I think that a lot of those tools can be repurposed and used for free for smaller organizations. And so my whole goal today is to put a few more tools in everybody's toolboxes. So we're gonna talk about three big things here. So the first is visualization, so that's the idea of taking any kind of data and turning it into pictures. Second is the idea of using context values. So numbers by themselves don't have meaning, but when a number has a story it has so much more power. And finally, segmentation, and that's just taking these numbers and putting human faces on them. They help people relate to them, and, and- just understand better, what's going on. So let's start out here with visualization, and I'm sure you guys are very familiar with this. We're gonna go back to that data we were just looking at, this is not really built for human brains, is it? You can empirically know the difference between 31 and 35, but when you look at a whole table of this stuff, I don't know about you guys, but my brain breaks down. And so visualization is really great for taking numbers like that, and making them more accessible to the way human brains are wired. And this is really, you know, ordinary data. This is just in the population of all 50 US states, but when you look at it, you can see things immediately that sort of jump out to you and your brain, because humans are good at, number one, recognizing patterns in numbers. Two, understanding spatial reasoning, and so you can see, hey, California has more people than Texas. And actually, to me, when I looked at this, it was kind of a surprising number. More, and you can see, proportionally, how few people are in Wyoming versus those more populous states. So, let's take this one step further, and talk about mapping. So this is the exact same data we were just looking at, but in the context of a map. And maps are an amazing way to display data, and I highly recommend using them whenever you possibly can, because people get maps. If-if you, you know, if you're not comfortable with math, but you probably still figured out how to use Google Maps to get someplace, so they're just better for people. They’re a really rich source of data, and they're very intuitive, and you can see, hey, look on the eastern half of the United States. That's probably where most people are, you know, except for Texas and California. And well, there's not a lot of people up in that north-central region, you get that at a glance, and I just want to take a second and talk about the data that I'm using here. So I've heard census come up a couple of times, the data I use a lot, it's called the American Community Survey data. The census happens every 10 years, it gets stale almost immediately. ACS comes out every single, January 2015 data just came out last January, and it's got literally thousands of variables, tons and tons of stuff, and it's free, it's paid for by your tax dollars, and all you have to do is use the American FactFinder to grab that data and use it for your grant applications or whatever you need. So moving on to our next topic, we're gonna talk about numbers, and context. So let me just say a number, 1.7 million. So the number by itself, you know, it sounds like a big number, but without a story behind it, it doesn't really mean a lot. So let me put a little bit of story behind that number. So 1.7 million Pennsylvania residents are disabled according to the American Community Survey, so that sounds like a big number right there, about 12 to 13 million people in Pennsylvania altogether, that seems like a good chunk of the residents. But really, is it? So let's talk about putting context via math on this stuff, and I know math can be a dirty word, but sometimes it's really good for making things simpler. So let's talk about indexes really quick, so this idea of using percentages, so we can look at the percentage of people disabled in Pennsylvania ends up being about 13.5 percent over the percentage of people disabled in the US. And this gives you an idea of an index, and you guys have probably heard of indexes before, so there's the stock index, there's the female economic empowerment index, so that compares the salaries of women to the salaries of men. So it's basically taking one thing that you want to look at, and comparing it to something else, to put it in context. So like I said, 13.5 percent of PA residents are disabled. So let's talk about a really specific example of indexes from the corporate world, just to sort of give you an idea of how this works in a lot of places. So indexes are used a lot, especially in advertising and marketing, and you guys might remember the show Mad Men. It ran on the network, AMC, they were able to charge the most money for ads of any television show when they were on for the last couple of seasons, and that's because of indexes. They over-indexed for households that made more than $100,000. So in other words, advertisers want to reach people who can afford their products, and so they look to target households that have more people who can afford those products, and so when a show over-indexes for that kind of person, they can charge more money per eyeball. And when you think about that in the context of nonprofits, it also can be really important. So you probably have an idea of you know when and where your donors come from, you probably have an idea of, you know, where the people you need to target are for your services, and indexes are a really easy way to understand that at a glance. So let's look at the, go back to this disabled example, so we've got 13.5 percent of Pennsylvania disabled, over twelve point four percent of the total United States that is disabled, so you can see the PA number is a little bit bigger. But how much bigger? So this is where indexes are really handy, and the thing I love about them is, you need to know one thing. Essentially, is it bigger than one, or less than one? And once you know that, you have an idea of what's going on, and sometimes, you know, like in this case, it's pretty close to one here. So you're like, “okay, there's more disabled people in Pennsylvania than in general, the United States, but it's pretty close. So you know, this is probably around on target, it's probably okay, but if it were eight or nine times more disabled people than the rest of the United States, you would probably look closer at Pennsylvania, and say, “hey, what's going on here?” And we're going to come back to this concept in a broader picture. So I'm gonna move on to our last topic which is segmentation. So segmentation is the idea of taking a broader population and breaking it down according to some factor that you think is important. And you guys probably have all have segments in your mind, you know exactly who you're going after, and again, to go back to the American Community Survey, there are a ton of data points in there that you can use to help figure out your segmentation, and figure out, okay, what's going on in different places. And sometimes, you can combine those segments. You can look for females aged 35 to 25 who live in households that don't have vehicles. And once you have that, it gets really, really powerful, because you can look at little slices of the public and figure out exactly what you need to do for each of them. So again, going back to the corporate world, I'm going to talk about Experian, which is a company out there. And they've something called mosaic segments, they cut the entire United States into 72 little pieces, and they charge a ton of money for this data. So I'm going to show you how to do it yourself so that you don't need to spend that kind of money. So let's just talk about some of their segments and what they look like so you can have an idea. So they take these segments, and they combine them into what's known as a persona, and so, what is a persona? A persona is almost like, it's like a mental stand-in for a person within that segment. And if you don't want it to be like really, really specific, cuz you don't wanna be making decisions about your program because Tim does not like ice cream. You want ‘em to be kinda broad, so here's an example of one of them. And this is at the very top of the mosaic segments, because you could tell it's important. Its income, and so it's the top one percent of US households. Generally the people in those households are over 50, and if you want to reach them in order to sell them something or talk to them, they have the latest tech. So it's generally the internet, or through their phones, that you reach them. Rooted flowerpower, this is the most prevalent mosaic group in my own neighborhood here in Pittsburgh, people tend to be over 60, they've been in their homes awhile there, they live a comfortable life, but they don't spend a lot of money. But they're one of the few segments still out there that will definitely watch TV commercials, and so if you want to reach them, you can actually get to them through their televisions, unlike some other people. And one more we're gonna talk about is diapers and debit cards. They tend to have service jobs that pay below the household income, they tend to live in apartments. They have one-to-three kids, but they live their whole lives for their mobile phones, so if you want to reach them through messaging, through their phones is the way to do it. So let's talk about how you can apply this to your own personal life. So the way that you build a persona is through interviews, so once you know what your segment is, and you want to interview, of course, more than one person. But you'd be surprised that you don't need to interview 100 people. Actually, six or seven will get you most of the way there. And once you've interviewed that many people, you'll see common threads. So the things they're saying, so I'll give you an example from my own life, I belong to an organization and they notice that the attendance for women was terrible. And it turned out that most of the women who wanted to be a member of the organization or were just not frequent attendees had kids. Whenever anything was scheduled in the evenings, you basically had negotiate with your spouse whether or not you could attend. You had to worry about child care. So they started offering free sitters as a part of their, as a part of their regular programming. And of course, the number of women attendees shot up. And they only found that by talking to people, and talking to more than one person. So let's let's just take one example to tie all this together. So this is Pennsylvania broken out by County. So we're going to talk about one specific type of Pennsylvania, which are high school students who live below the poverty line. And you might notice that there are more than 12 counties in Pennsylvania, but there are only six highlighted here that over-index for the number of high school students who live below the poverty line. And that's because of that County down there in the southeastern corner of the Pennsylvania, that is Philadelphia County, which has by far the most high school students in poverty in the entire state. And because it's such a large district, it drags everything down. Now the number of students in poverty is actually not too far away from the the actual state average, it's, I think, the index is about 1.25. But the one that's really astounding is, over there in the northeastern part of the state, that is Cameron County, twice the number of the state average of high school students that lived below the poverty line. So if you're an organization, and you're trying to figure out where in Pennsylvania do I target, indexes are a great way of showing where your crisis spots are, and we're to figure out where to go first. So this sort of brings that whole picture together, and once you have that then, you can start interviewing people, building personas, and then designing what your offerings are gonna be. So I really want to thank you guys for having me here today, we're gonna be outside in the booth, so if you want to know more about data in general, or, you know, how you can use mapping data for your organization, we're gonna be right outside. Thank you so much for having us, it's been a pleasure being here, it's been awesome meeting you all, you guys. Thank you.